In 2025, I resolve to eliminate escalations and finger pointing
Steffen Geißinger
January 14, 2025
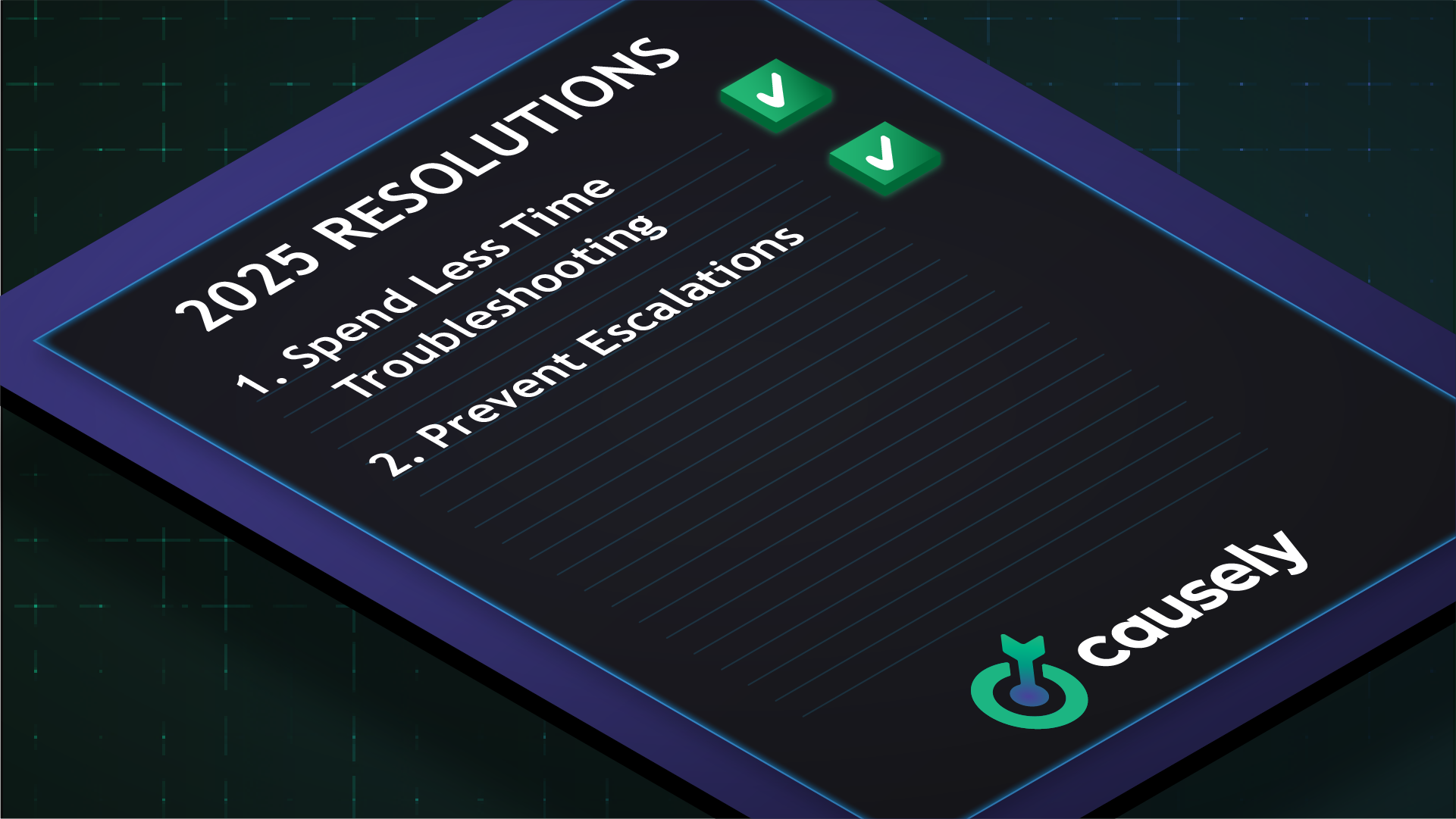
Make escalations less about blame and more about progress
Microservices architectures introduce complex, dynamic dependencies between loosely coupled components. In turn, these dependencies lead to complex, hard to predict interactions. In these environments, any resource bottleneck, or any service bottleneck or malfunction, will cascade and affect multiple services, crossing team boundaries. As a result, the response often spirals into a chaotic mix of war rooms, heated Slack threads, and finger-pointing. The problem isn’t just technical—it’s structural. Without a clear understanding of dependencies and ownership, every team spends more time defending their work than solving the issue. It’s a waste of effort that undermines collaboration and prolongs downtime.
Yesterday, we resolved to spend less time troubleshooting in 2025.
Troubleshooting and escalation are closely intertwined. A single unresolved bottleneck can ripple outward, forcing multiple teams into reactive mode as they struggle to isolate the true root cause. This dynamic creates inefficiencies and delays, with teams often focusing on band-aiding symptoms instead of remediating and solving the root causes. To eliminate this friction, we need systems that do more than detect anomalies—they must provide a seamless view of dependencies, understand and analyze the performance behaviors of the microservices, assign ownership intelligently, and guide engineers toward resolution with precision and context.
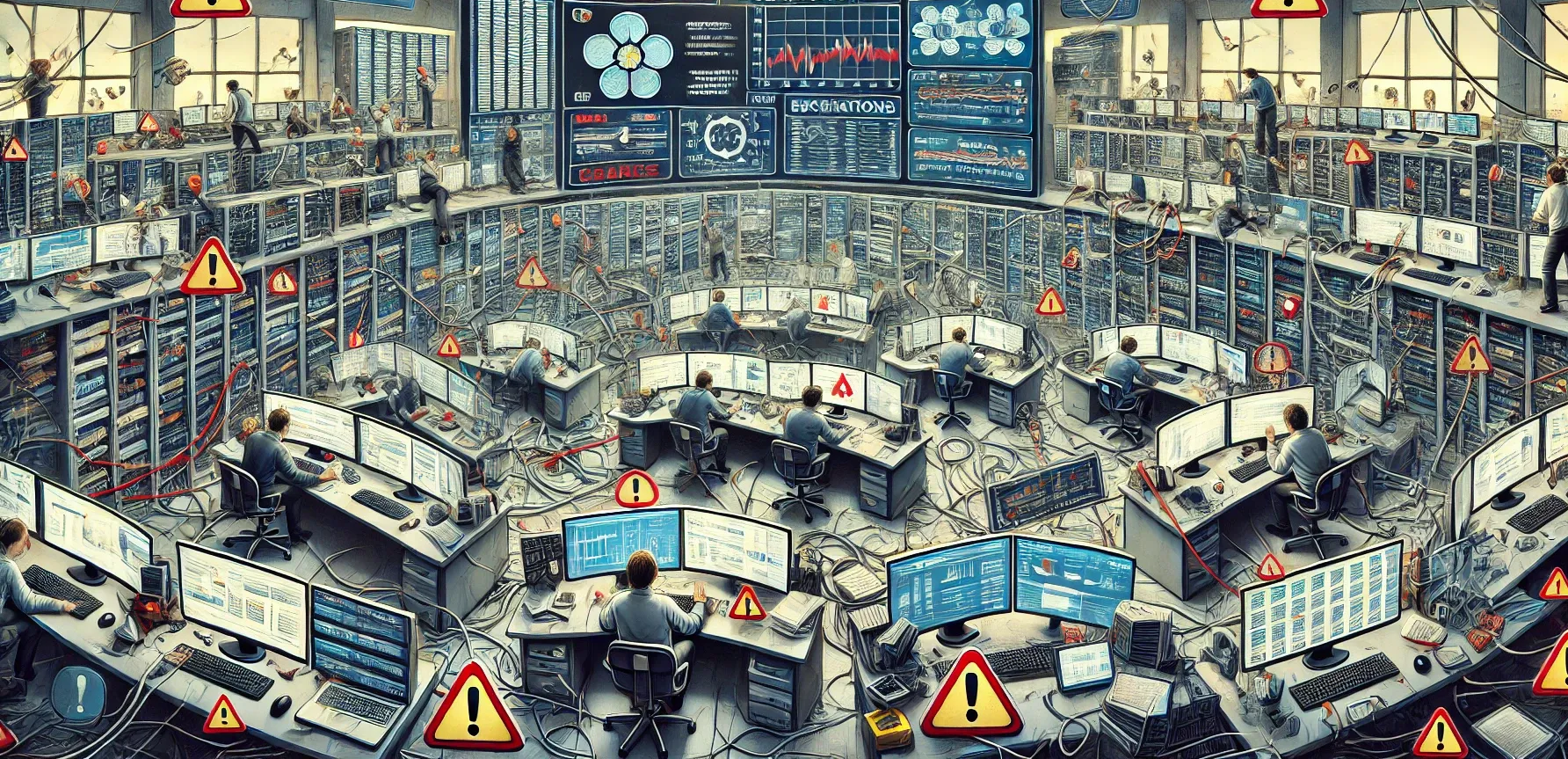
Take, for example, an application developer who notices high request duration for users who are trying to interact with their application. This application communicates with many different services, and it happens to run within a container environment on public cloud infrastructure. There are more than 50 possible root causes that might be causing the high request duration issue. That developer would need to investigate garbage collection issues, disk congestion, app-locking problems, and node congestion among many other potential root causes until accurately determining that a congested database is the source of their problem. The only proper way to determine root cause is by considering all the cause-and-effect relationships between all the possible root causes and the symptoms they may cause. This process can often take hours or days before the correct root cause is pinpointed, resulting in a variety of business consequences (unhappy users, missed SLOs, SLA violations, etc.).
In this post, we’ll explore the challenges of multi-team escalations, and the capabilities needed to address them. From automated dependency mapping to explainable triage workflows, we’ll show how observability can be transformed from chaos into clarity, making escalations less contentious and far more productive.
Escalations can cripple teams
Escalations create inefficiencies that extend downtime, frustrate teams, and waste resources. These inefficiencies stem from a combination of structural and technical gaps in how dependencies are understood, root causes are isolated, and ownership is assigned. Here are some of the key challenges that make escalations so painful today:
- There is a lack of cross-team visibility into dependencies
- It can be hard to predict or analyze the performance behaviors of loosely coupled dependent microservices
- It can be difficult to isolate the root cause among all affected services
- Legacy observability tools must be stitched together to provide even partial visibility into issues
Lack of cross-team visibility
Microservices architectures are complex and full of deeply interconnected components. An issue in one can cascade into others. Without clear visibility into these dependencies, teams are left guessing which components are impacted and which team should take ownership.
Your favorite observability tools help you visualize dependencies, but they lack real-time accuracy. These maps can quickly become outdated in environments with frequent changes. Some of them are great for aggregating logs, but don’t offer much insight into service relationships. Engineers are often left to piece together dependencies manually.
Unpredictable performance behavior of microservices
Loosely coupled microservices communicate with each other and share resources. But which services depend on which? And what resources are shared by which services? These dependencies are continuously changing and, in many cases, unpredictable.
A congested database may cause performance degradations of some services that are accessing the database. But which one will be degraded? Hard to know. Depends. Which services are accessing which tables through what APIs? Are all tables or APIs impacted by the bottleneck? Which other services depend on the services that are degraded? Are all of them going to be degraded? These are very difficult questions to answer.
As a result, predicting, understanding and analyzing the performance behavior of each service is very difficult. Using existing brittle observability tools to diagnose how a bottleneck cascades across services is practically impossible.
Difficulty identifying root causes among all affected services
Determining what’s a cause and what’s a symptom can be an incredibly time-consuming aspect of troubleshooting and escalations. Further, the person or team identifying a problem may well be looking at only their local maxima: the part of the system they work on or are directly affected by. They often don’t see the full picture of all intertwined systems. Identifying the root cause among all affected services can be inordinately difficult.
Even if you have tools that are excellent for visualizing time-series data, you must still rely on engineers to manually correlate metrics. APM tools can help you examine application performance but require significant manual effort to link symptoms to underlying causes, especially in microservices-based, cloud-native applications.
Legacy observability tooling only gives you partial functionality
While both established and up-and-coming tools offer valuable capabilities, they often address only one part of the problem, leaving critical gaps. Dependency visibility, performance analysis and root cause isolation need to be integrated seamlessly to reduce the chaos of escalations. Today’s tools, however, are fragmented, requiring engineers to bridge the gaps manually, costing valuable time and effort during incidents. Solving these problems demands a holistic approach that ties all these elements together in real time.
How escalations should be handled
Escalations have negative consequences for organizations of all sizes. Let’s work together to build systems that render escalations less about blame and more about opportunities to foster trust and collaboration. These systems will have the following capabilities:
- End-to-end discovery of service dependencies. Automatically discover and maintain, in real time, a complete view of how systems interact.
- Workflow integration that directs root cause resolution to the correct team. Use the tools your organization has already invested in to turn root causes into actions for the correct team, reducing delays caused by miscommunication.
- Performance analysis of bottlenecks propagations. Provide insights on how bottlenecks cascade across services.
- Detailed identification of root causes across an entire system. Empower engineers to act confidently without over-reliance on senior team members.
With these new systems, escalations can result in positive business outcomes:
- Deep, real-time understanding of complete microservices systems
- Better collaboration between teams to remediate issues as they arise
- More innovation, less time in war rooms
- Empowered engineering teams that solve problems instead of pointing fingers
Causely helps you handle escalations quickly and confidently
Our Causal Reasoning Platform is a model-driven, purpose-built AI system delivering multiple analytics built on a common data model. It includes several features to help you understand issues and handle escalations efficiently:
- Out-of-the-box Causal Models. Causely is delivered with built-in causality knowledge capturing the common root causes that can occur in cloud-native environments. This causality knowledge enables Causely to automatically pinpoint root causes out-of-the-box as soon as it is deployed in an environment. There are at least a few important details to share about this causality knowledge:
- It captures potential root causes in a broad range of entities including applications, databases, caches, messaging, load balancers, DNS, compute, storage, and more.
- It describes how the root causes will propagate across the entire environment and what symptoms may be observed when each of the root causes occurs.
- It is completely independent from any specific environment and is applicable to any cloud-native application environment.
- Automatic topology discovery. Cloud-native environments are a tangled web of applications and services layered over complex and dynamic infrastructure. Causely automatically discovers all the entities in the environment including the applications, services, databases, caches, messaging, load balancers, compute, storage, etc., as well as how they all relate to each other. For each discovered entity, Causely automatically discovers its:
- Connectivity - the entities it is connected to and the entities it is communicating with horizontally
- Layering - the entities it is vertically layered over or underlying
- Composition - what the entity itself is composed of
Causely automatically stitches all of these relationships together to generate a Topology Graph, which is a clear dependency map of the entire environment. This Topology Graph updates continuously in real time, accurately representing the current state of the environment at all times.
- Root cause analysis. Using the out-of-the-box Causal Models and the Topology Graph as described above, Causely automatically generates a causal mapping between all the possible root causes and the symptoms each of them may cause, along with the probability that each symptom would be observed when the root cause occurs. Causely uses this causal mapping to automatically pinpoint root causes based on observed symptoms in real time. No configuration is required for Causely to immediately pinpoint a broad set of root causes (100+), ranging from applications malfunctioning to services congestion to infrastructure bottlenecks.
In any given environment, there can be tens of thousands of different root causes that may cause hundreds of thousands of symptoms. Causely prevents SLO violations by detangling this mess and pinpointing the root cause putting your SLOs at risk and driving remediation actions before SLOs are violated. For example, Causely proactively pinpoints if a software update changes performance behaviors for dependent services before those services are impacted. - Performance analysis. Causely analyzes microservices performance bottleneck propagation by automatically learning, based on your data:
- the correlation between the loads on services, i.e., how a change in load of one cascades and impacts the loads on other services;
- the correlation between services latencies, i.e., how latency of one cascades and impacts the latencies of other services; and
- the likelihood a service or resource bottleneck may cause performance degradations on dependent services.
- Constraints analysis. Causely uses performance goals like throughput and latency, and capacity or cost constraints, and automatically figures out what actions need to be taken to assure the goals are accomplished while satisfying the constraints.
- Prevention analysis. Teams can also ask "what if'' questions to understand the impact that potential problems might have if they were to occur to support the planning of service/architecture changes, maintenance activities, and service resiliency improvements.
- Predictive analysis. Causely automatically analyzes performance trends and pinpoints the actions required to prevent future degradations, SLO violations, or constraints.
- Service impact analysis. Causely automatically analyzes the impact of the root causes on SLOs, prioritizing the root causes based on the violated SLOs and those that are at risk. Causely automatically defines standard SLOs (based on latency and error rate) and uses machine learning to improve its anomaly detection over time. However, environments that already have SLO definitions in another system can easily be incorporated in place of Causely’s default settings.
- Contextual presentation. Results are intuitively presented in the Causely UI, enabling users to see the root causes, related symptoms, the service impacts and initiate remedial actions. The results can also be sent to external systems to alert teams who are responsible for remediating root cause problems, to notify teams whose services are impacted, and to initiate incident response workflows.
- Postmortem analysis. Teams can also review prior incidents and see clear explanations of why these occurred and what the effect was, simplifying the process of postmortems, enabling actions to be taken to avoid re-occurrences.
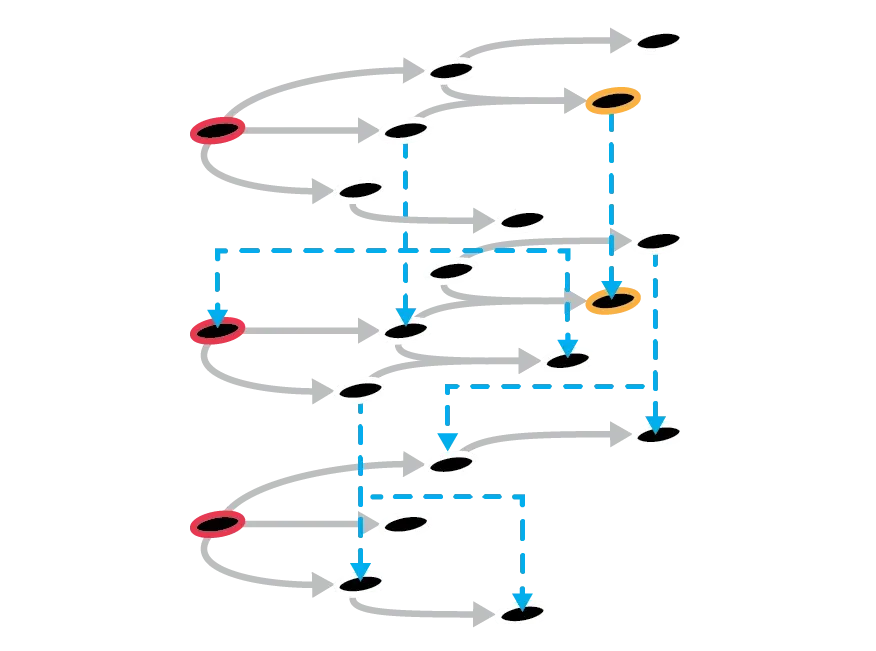
Conclusion
Escalations don’t need to devolve into chaos, finger pointing, and frayed relationships. They can be opportunities for teams to solve real problems together. The key is having dependable, real-time information on service dependencies and root causes of problems. Armed with the right information, teams can work efficiently and collaboratively to maintain system reliability.
Book a meeting with the Causely team and let us show you how to transform the state of escalations and cross-organizational collaboration in cloud-native environments.