Fools Gold or Future Fixer: Can AI-powered Causality Crack the RCA Code for Cloud Native Applications?
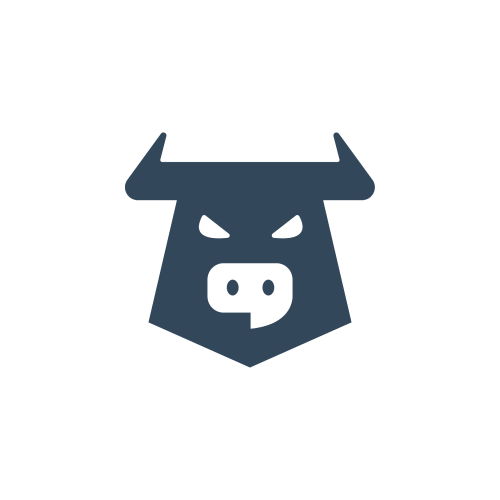
Causely
April 8, 2024
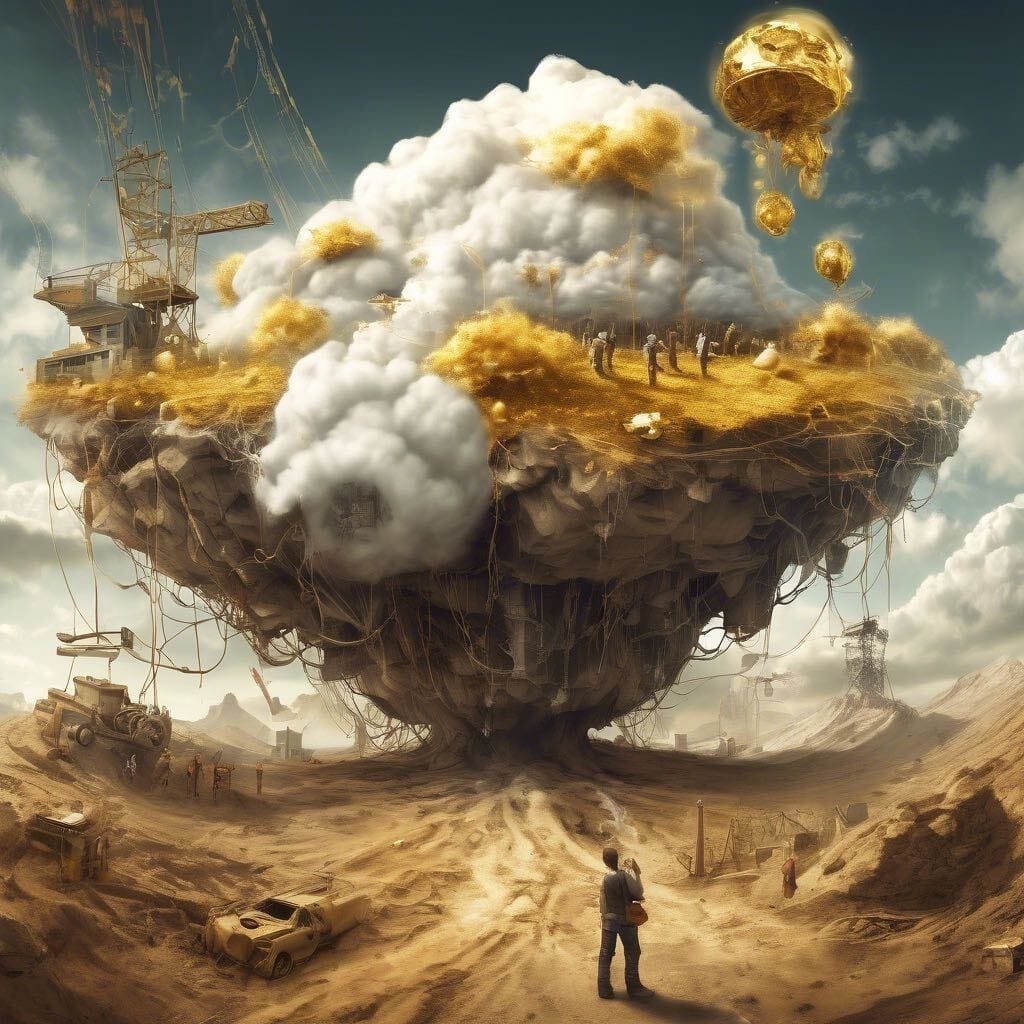
The idea of applying AI to determine causality in an automated Root Cause Analysis solution sounds like the Holy Grail, but it’s easier said than done. There’s a lot of misinformation surrounding RCA solutions. This article cuts the confusion and provides a clear picture. I will outline the essential functionalities needed for automated root cause analysis. Not only will I define these capabilities, I will also showcase some examples to demonstrate their impact.
By the end, you’ll have a clearer understanding of what a robust RCA solution powered by causal AI can offer and how it can empower your IT team to better navigate the complexities of your cloud-native environment and most importantly dramatically reduce MTTx.
The Rise (and Fall) of the Automated Root Cause Analysis Holy Grail
Modern organizations are tethered to technology. IT systems, once monolithic and predictable, have fractured into a dynamic web of cloud-native applications. This shift towards agility and scalability has come at a cost: unprecedented complexity.
Troubleshooting these intricate ecosystems is a constant struggle for DevOps teams. Pinpointing the root cause of performance issues and malfunctions can feel like navigating a labyrinth – a seemingly endless path of interconnected components, each with the potential to be the culprit.
For years, automating Root Cause Analysis (RCA) has been the elusive “Holy Grail” for service assurance, as the business consequences of poorly performing systems are undeniable, especially as organizations become increasingly reliant on digital platforms.
Despite its importance, commercially available solutions for automated RCA remain scarce. While some hyperscalers and large enterprises have the resources and capital to attempt to develop in-house solutions to address the challenge (like Capital One’s example), these capabilities are out of reach for most organizations.
See how Causely can help your organization eliminate human troubleshooting. Request a demo of the Causal AI platform.Beyond Service Status: Unraveling the Cause-and-Effect Relations in Cloud Native Applications
Highly distributed systems, regardless of technology, are vulnerable to failures that cascade and impact interconnected components. Cloud-native environments, due to their complex web of dependencies, are especially prone to this domino effect. Imagine a single malfunction in a microservice, triggering chain reaction, disrupting related microservices. Similarly, a database issue can ripple outwards, affecting its clients and in turn everything that relies on them.
The same applies to infrastructure services like Kubernetes, Kafka, and RabbitMQ. Problems in these platforms might not always be immediately obvious because of the symptoms they cause within their domain. Furthermore symptoms manifest themselves within applications they support. The problem can then propagate further to related applications, creating a situation where the root cause problem and the symptoms they cause are separated by several layers.
Although many observability tools offer maps and graphs to visualize infrastructure and application health, these can become overwhelming during service disruptions and outages. While a sea of red icons in a topology map might highlight one or more issues, they fail to illuminate cause-and-effect relationships. Users are then left to decipher the complex interplay of problems and symptoms to work out the root cause. This is even harder to decipher when multiple root causes are present that have overlapping symptoms.
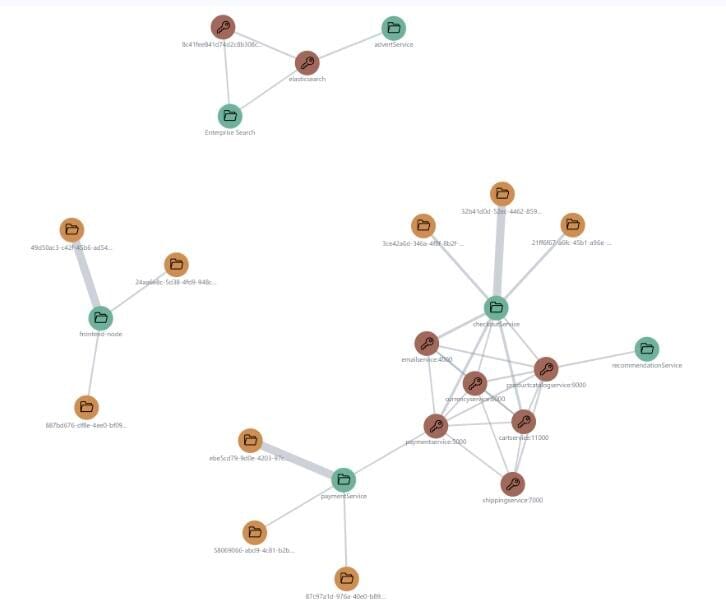
In addition to topology based correlation, DevOps team may also have experience of other types of correlation including event deduplication, time based correlation and path based analysis all of which attempt to reduce the noise in observability data. Don’t loose sight of the fact that this is not root cause analysis, just correlation, and correlation does not equal causation. This subject is covered further in a previous article I published Unveiling The Causal Revolution in Observability.
The Holy Grail of troubleshooting lies in understanding causality. Moving beyond topology maps and graphs, we need solutions that represent causality depicting the complex chains of cause-and-effect relationships, with clear lines of responsibility. Precise root cause identification that clearly explains the relationship between root causes and the symptoms they cause, spanning the technology domains that support application service composition, empowers DevOps teams to:
- Accelerate Resolution: By pinpointing the exact source of the issue and the symptoms that are caused by this, responsible teams are notified instantly and can prioritize fixes based on a clear understanding of the magnitude of the problem. This laser focus translates to faster resolution times.
- Minimize Triage: Teams managing impacted services are spared the burden of extensive troubleshooting. They can receive immediate notification of the issue’s origin, impact, and ownership, eliminating unnecessary investigation and streamlining recovery.
- Enhance Collaboration: With a clear understanding of complex chains of cause-and-effect relationships, teams can collaborate more effectively. The root cause owner can concentrate on fixing the issue, while impacted service teams can implement mitigating measures to minimize downstream effects.
- Automate Responses: Understanding cause and effect is also an enabler for automated workflows. This might include automatically notifying relevant teams through collaboration tools, notification systems and the service desk, as well as triggering remedial actions based on the identified problem.
Bringing This to Life with Real World Examples
The following examples will showcase the concept of causality relations, illustrating the precise relationships between root cause problems and the symptoms they trigger in interrelated components that make up application services.
This knowledge is crucial for several reasons. First, it allows for targeted notifications. By understanding the cause-and-effect sequences, the right teams can be swiftly alerted when issues arise, enabling faster resolution. Second, service owners impacted by problems can pinpoint the responsible parties. This clarity empowers them to take mitigating actions within their own services whenever possible and not waste time troubleshooting issues that fall outside of their area of responsibility.
Infra Problem Impacting Multiple Services
In this example, a CPU congestion in a Kubernetes Pod is the root cause and this causes symptoms – high latency – in application services that it is hosting. In turn, this results in high latency on other applications services. In this situation the causal relationships are clearly explained.
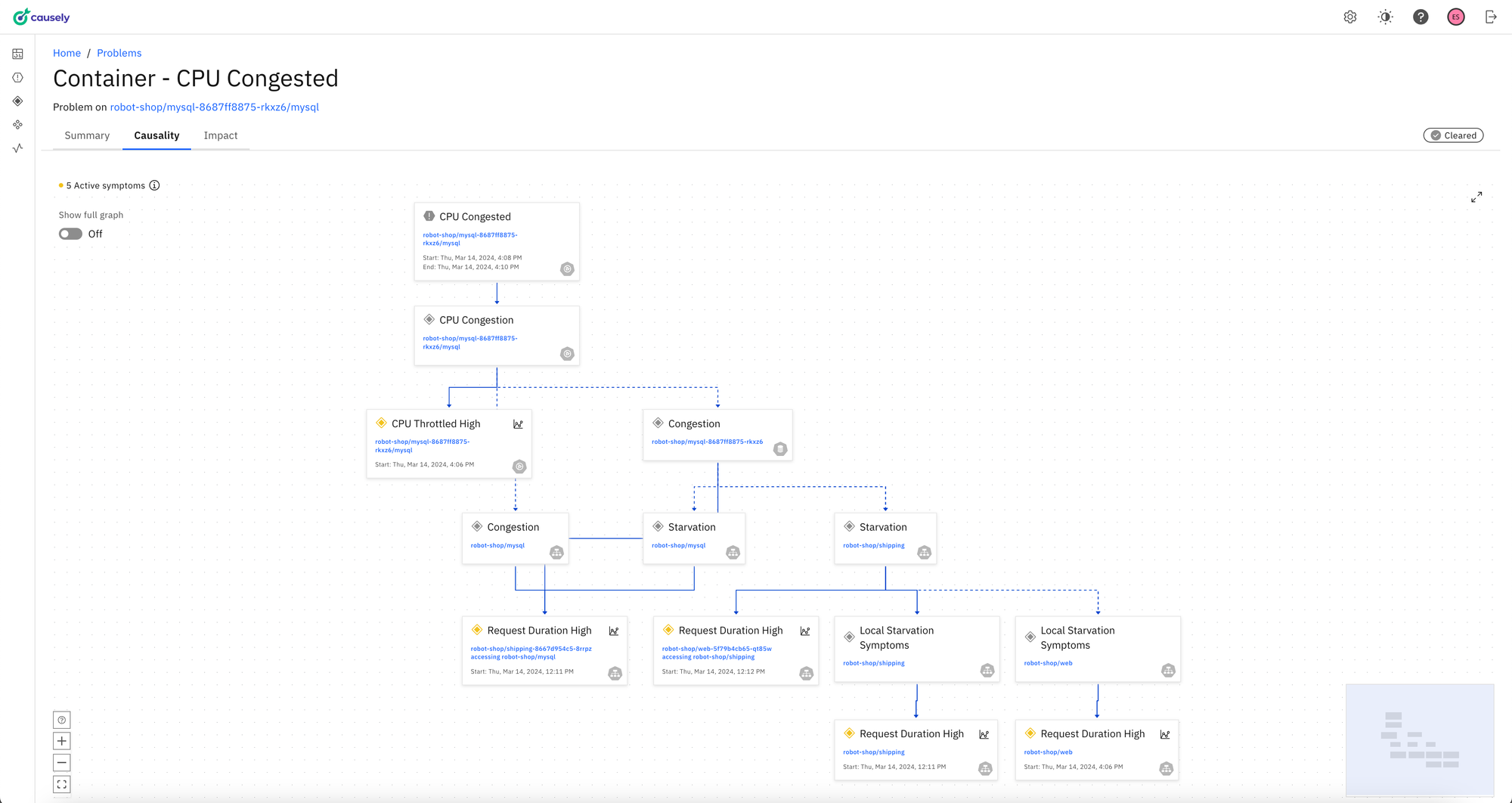
A Microservice Hiccup Leads to Consumer Lag
Imagine you’re relying on a real-time data feed, but the information you see is outdated. In this scenario, a bug within a microservice (the data producer) disrupts its ability to send updates. This creates a backlog of events, causing downstream consumers (the services that use the data) to fall behind. As a result, users/customers end up seeing stale data, impacting the overall user experience and potentially leading to inaccurate decisions. Very often the first time DevOps find out about these types of issues is when end users and customers complain about the service experience.
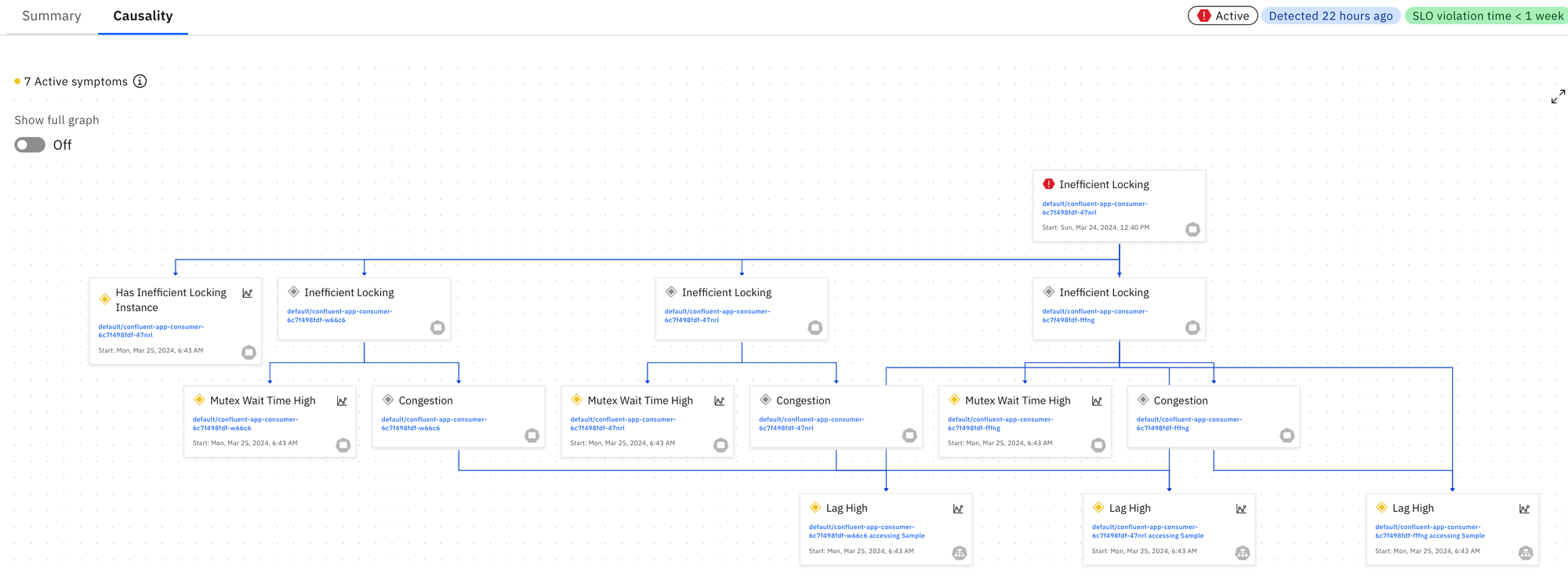
Database Problems
In this example the clients of a database are experiencing performance issues because one of the clients is issuing queries that are particularly resource-intensive. Symptoms of this include:
- Slow query response times: Other queries submitted to the database take a significantly longer time to execute.
- Increased wait times for resources: Applications using the database experience high error rate as they wait for resources like CPU or disk access that are being heavily utilized by the resource-intensive queries.
- Database connection timeouts: If the database becomes overloaded due to the resource-intensive queries, applications might experience timeouts when trying to connect.
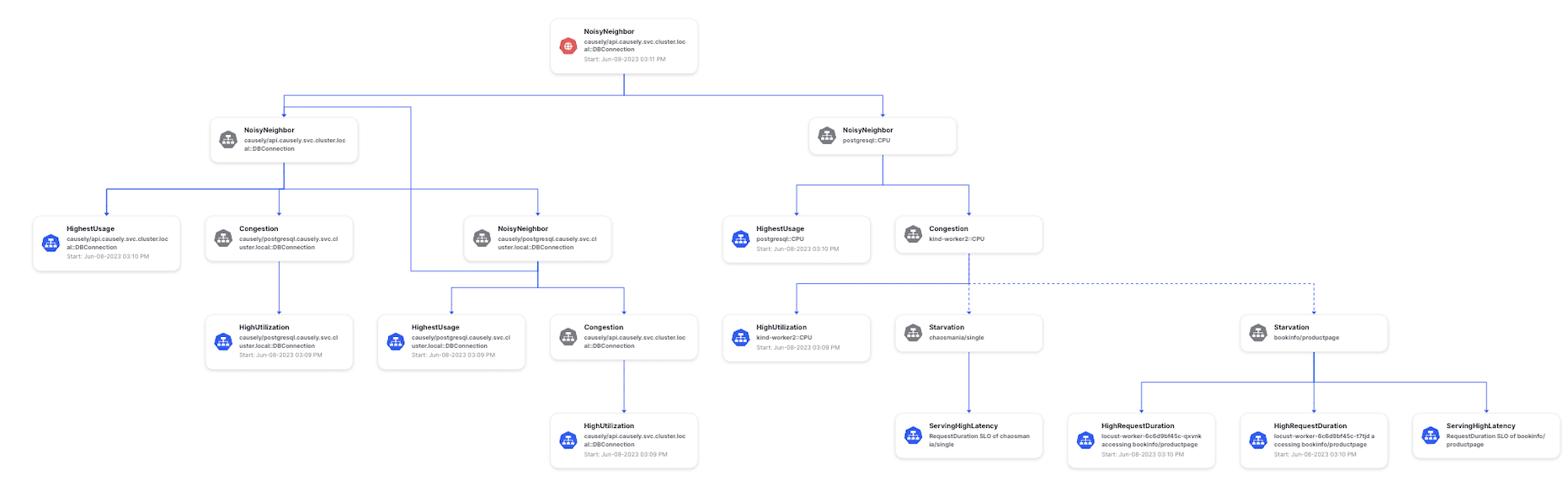
Summing Up
Cloud-native systems bring agility and scalability, but troubleshooting can be a nightmare. Here’s what you need to conquer Root Cause Analysis (RCA) in this complex world:
- Automated Analysis: Move beyond time-consuming manual RCA. Effective solutions automate data collection and analysis to pinpoint cause-and-effect relationships swiftly.
- Causal Reasoning: Don’t settle for mere correlations. True RCA tools understand causal chains, clearly and accurately explaining “why” things happen and the impact that they have.
- Dynamic Learning: Cloud-native environments are living ecosystems. RCA solutions must continuously learn and adapt to maintain accuracy as the landscape changes.
- Abstraction: Cut through the complexity. Effective RCA tools provide a clear view, hiding unnecessary details and highlighting crucial troubleshooting information.
- Time Travel:Post incident analysis requires clear explanations. Go back in time to understand “why” problems and understand the impact they had.
- Hypothesis: Understand the impact that degradation or failures in application services and infrastructure will have before they happen.
These capabilities unlock significant benefits:
- Faster Mean Time to Resolution (MTTR): Get back to business quickly.
- More Efficient Use Of Resources: Eliminate wasted time chasing the symptoms of problems and get to the root cause immediately.
- Free Up Expert Resources From Troubleshooting: Empower less specialized teams to take ownership of the work.
- Improved Collaboration: Foster teamwork because everyone understands the cause-and-effect chain.
- Reduced Costs & Disruptions: Save money and minimize business interruptions.
- Enhanced Innovation & Employee Satisfaction: Free up resources for innovation and create a smoother work environment.
- Improved Resilience: Take action now to prevent problems that could impact application performance and availability in the future
If you would like to get to avoid the glitter of “Fools Gold” and get to the Holy Grail of service assurance with automated Root Cause Analysis don’t hesitate to reach out to me directly, or contact the team at Causely today to discuss your challenges and discover how they can help you.